Ziyang Wang
Ph.D. Candidate at Rice University
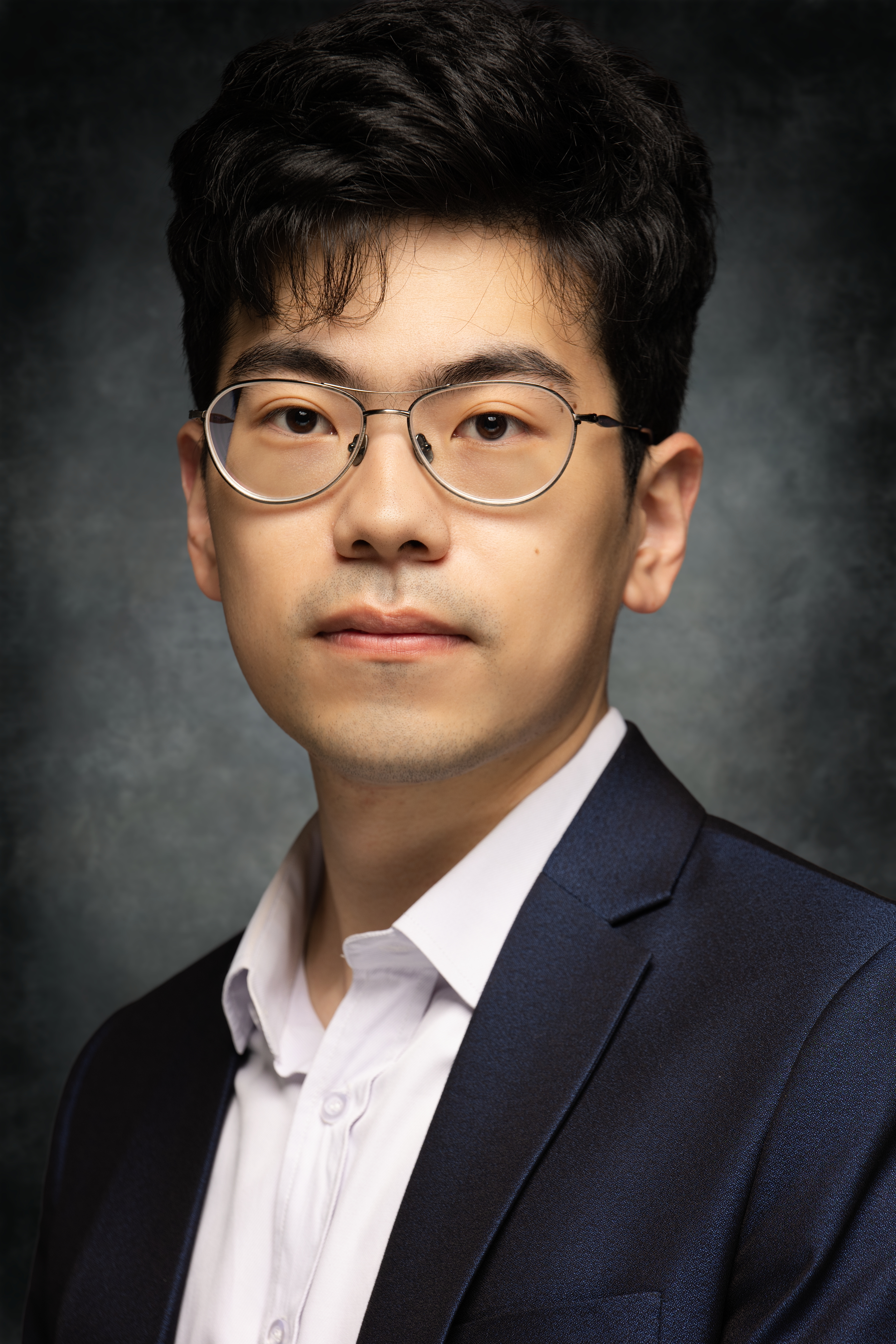
I am a fifth-year Ph.D. student in Electrical and Computer Engineering at Rice University, with a background as a Schreyer Honors Scholar majoring in Computer Science and Mathematics from The Pennsylvania State University. I am currently a member of SCOPE lab, advised by Prof. Shengxi Huang and work closely with Prof. Yuxuan ‘Cosmi’ Lin. My research lies at the intersection of artificial intelligence (AI), computational science, and experimental technology, focusing on developing machine learning (ML) methodologies to advance biomedical sciences, materials research, and molecular sensing.
My work has contributed to AI-driven innovations in disease diagnosis, biomarker discovery, virus identification, cancer research, and next-generation material characterization. My vision is to leverage ML to enhance scientific instruments, enable automated experiments, and accelerate high-accuracy simulations in biomedical and material sciences. I explore cross-disciplinary applications in food safety, agricultural monitoring, and environmental science to expand ML’s impact across diverse domains and translate these advancements into real-world solutions. My goal is to unlock ML’s transformative potential in scientific discovery, ultimately fostering breakthroughs that improve human health and well-being.
In addition to my academic pursuits, I am a co-founder of iDeal technology LLC and developed a mobile app on Android and IOS helping community rentals and trading. These experiences have honed my skills in entrepreneurship and leadership, allowing me to apply theoretical knowledge to practical solutions.
selected publications
- ACS NanoRapid biomarker screening of Alzheimer’s disease by interpretable machine learning and graphene-assisted Raman spectroscopyACS nano, 2022
- 2D MaterialsMeasuring complex refractive index through deep-learning-enabled optical reflectometry2D Materials, 2023
- ACS photonicsUnderstanding the excitation wavelength dependence and thermal stability of the SARS-CoV-2 receptor-binding domain using surface-enhanced raman scattering and machine learningACS photonics, 2022
- PNASAccurate virus identification with interpretable Raman signatures by machine learningProceedings of the National Academy of Sciences, 2022